Need, Approach, Benefit, Competition
The increasing volume of text datasets is challenging the cognitive capabilities of expert analysts to produce meaningful insights. Large-scale distributed agents like machine learning algorithms and crowd workers present new opportunities to make sense of big data. However, we must first overcome the challenge of modeling and guiding the overall process so that many distributed agents can meaningfully contribute to suitable components. Inspired by the sensemaking loop, collaboration models, and investigation techniques used in Intelligence Analysis community, we propose a pipeline to better enable collaboration among expert analysts, crowds, and algorithms. We modularize and clarify the components in the sensemaking loop so that they are connected via clearly defined inputs and outputs to pass intermediate analysis results along the pipeline, and can be assigned to different agents with appropriate techniques. We instantiate the pipeline with a location-based investigation strategies and recruited 134 crowd workers on Amazon Mechanical Turk to analyze the dataset. Our results show that the pipeline can successfully guide crowd workers to contribute meaningful insights that are helpful to solve complicated sensemaking challenges. This allows us to imagine broader possibilities for how each component could be executed: with individual experts, crowds, or algorithms, as well as new combinations of these, where each is best suited.
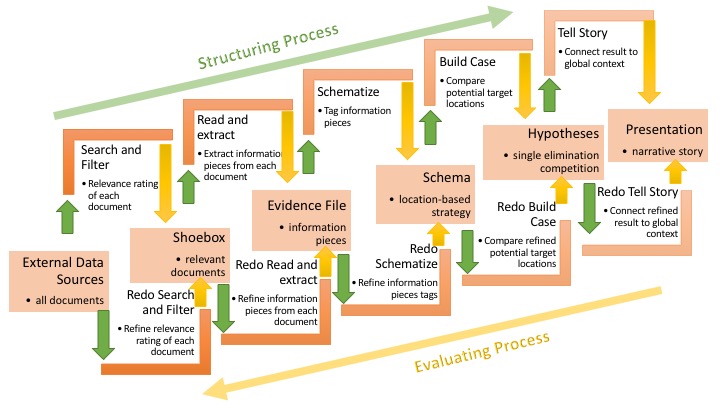
Result and Discussion
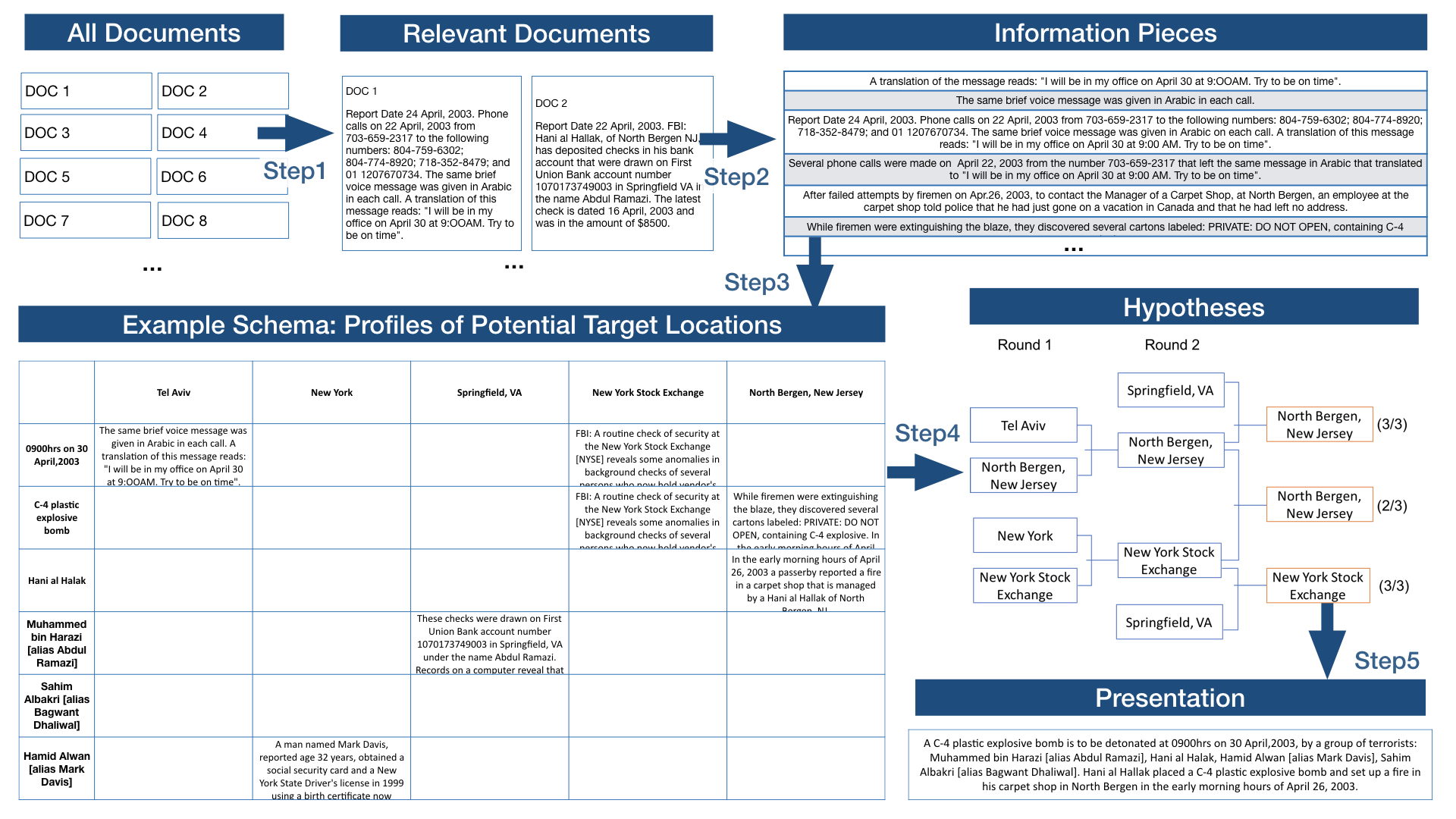
Step1: Subtly Relevant Documents are Successfully Retrieved
7 documents out of 13 directly mentioned one or more key elements. The remaining 6 documents (3 relevant and 3 irrelevant) are rated by crowd workers in a 0-100 scale. A neutral threshold 50 leads to 11 documents as Step 1 Data Output (precision=90.1%, recall=100%).
Step2: Most Key Useful Information Pieces are Extracted
In Step 2, a total of 26 information pieces are extracted. 18 of the information pieces mentions the target location and the key elements. However, some important information pieces didn’t get extracted.
Step3: Accurate Tagging and Potential Target Identification
After Step 3, 18 out of all the 26 information pieces are tagged, including 5 location tags. One interesting finding is two different workers both identified a location “Tel Aviv” in the information piece “The same brief voice message was given in Arabic in each call. A translation of this message reads: “I will be in my office on April 30 at 9:OOAM. Try to be on time”. One of the workers even give very specific information “the lacation (location) is israel (Israel) at “Mike’s Place”, a restaurant in Tel Aviv”. This means the crowds connect their own knowledge to this analysis.
Step4: Reasonable Reasoning and Comparison
For each location recognized in Step 3, we organize the source information piece to form the profile of this location tag. Then we rank the locations by amount of evidence to prepare for a single-elimination competition. The final winning location is “North Bergen, New Jersey” (the last place the bomb was stored before transferred to the target location), the second place is New York Stock Exchange (the real answer), losing with only one vote.
Logical and Clear Narrative Presentation
Using the profile of “North Bergen, New Jersey”, workers created a narrative as follows:
“A C-4 plastic explosive bomb is to be detonated at 0900hrs on 30 April,2003, by a group of terrorists: Muhammed bin Harazi [alias Abdul Ramazi], Hani al Halak, Hamid Alwan [alias Mark Davis], Sahim Albakri [alias Bagwant Dhaliwal]. Hani al Hallak placed a C-4 plastic explosive bomb and set up a fire in his carpet shop in North Bergen in the early morning hours of April 26, 2003.”