[1]. MITRA, T.; COUNTS, S.; PENNEBAKER, J.. Understanding Anti-Vaccination Attitudes in Social Media. International AAAI Conference on Web and Social Media, North America, mar. 2016.
[2]. Choudhury, M.D., Counts, S., Gamon, M., & Horvitz, E. (2013). Predicting Depression via Social Media. ICWSM.
Summaries:
In [1] Mitra et al. tried to figure out the anti-vaccination attitudes in social media. The authors tried to understand the attitudes of participants involved in the vaccination debate on Twitter. They used five phrases related to vaccines to gather data from January 01, 2012 to June 30, 2015, totaling to 315,240 tweets generated by 144,817 unique users. After filtering, the final dataset had 49,354 tweets by 32,282 unique users. These users were classified into three groups: pro-vaccine, anti-vaccine and joining-anti (those who convert to anti-vaccination). The authors found that the long-term anti-vaccination supporters have conspiratorial views and are firm in their beliefs. Also, the “joining-anti” share similar conspiracy thinking but they tend to be less assured and more social in nature.
In [2] Choudhury et al. predict depression via social media. The authors use crowdsourcing to compile a set of Twitter users who report being diagnosed with clinical depression, based on a standard psychometric instrument. The total of 1,583 crowd-workers completed the human intelligence tasks and only 637 participants provided the access to their Twitter feeds. After filtering, the final dataset had 476 users who self-reported to have been diagnosed with depression in the given time range. They measured the behavioral attributes relating to social engagement, emotion, language and linguistic styles, ego network, and mentions of antidepressant medications of these 476 users over a year preceding the onset of depression. They built a statistical classifier that provides estimates of the risk of depression before the reported onset with an average accuracy of ~70%. The authors found that the individuals with depression show lowered social activity, greater negative emotion and much greater medicinal concerns and religious thoughts.
Reflections:
Both the papers are very socially relevant and I really enjoyed both readings. The first paper says that the long-term anti-vaccination supporters are very firm in their conspiracy views and we need new tactics to counter the damaging consequences of anti-vaccination beliefs but I think that the paper missed a very key analysis of fourth class of people “joining-pro”. Analyzing the fourth class might have provided some key insights into “tactics” to counter anti-vaccination beliefs. I also have major concerns regarding the data preparation. Even though MMR is related to vaccines but autism has more to do with genes because autism tends to run in families which makes me question that why did 3 out of 5 phrases had autism is it? Secondly, the initial dataset to identify pro and anti stance contained 315,240 tweets generated by 144,817 individuals and the final dataset had 49,354 tweets by 32,282 unique users. This means that each user on average had 1.5 tweets relating to vaccines in almost 3.5 years. Is this data enough to classify the users as pro-vaccination or anti-vaccination? Because millions of tweets of these same users are analyzed in the analysis.
The second paper was also an excellent read and even though 70% accuracy might not be the most desirable results in this case but I really liked the way entire analysis was conducted. The authors mined 10% sample of a snapshot of the “Mental Health” category of Yahoo! Answers. One thing that I would like to know is that is mining websites ethical? Because scraping often violates the terms of service of the target website and secondly, publishing scraped content may be a breach of copyright. I also have a doubt that how can all “randomly” selected 5 posts in Table 2 out of 2.1 million tweets be all exactly related to depression. Was this at all really random?
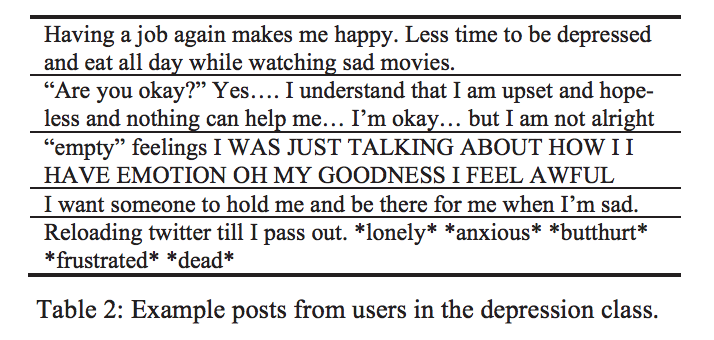
I also feel that the third post has more to do with sarcasm than depression. Which makes me wonder why sarcasm was not catered for in the analysis?
Furthermore, just like the first paper, I have concerns with the data collection for this paper as well. They had put me off when they said that they paid 90 cents for completing the tasks. Is everyone really going to fill anything with complete honesty for 90 cents? Secondly, 476 out of final 554 users self-reported to have been diagnosed with depression, which is ~86% of users where as, the depression rate is US is ~16%. Which makes me question again that did they fill the survey honestly, especially if you are paying them 90 cents? Other than that, the authors did a terribly good job in analysis especially the features that they created. They covered all grounds from number of inlinks to linguistic styles to even the use of antidepressants. I believe that except for the data part, the analysis of both papers were thorough done and are excellent examples of good experimental designs.
Read More