Does human autonomy have a place in discussions of algorithmic fairness?
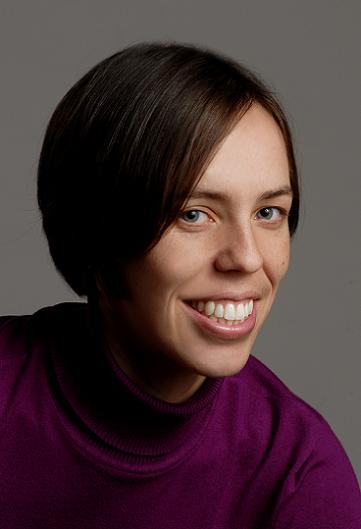
Research in the area of algorithmic ‘fairness’ often leans toward narrower definitions of fairness that can be subject to formalism and implementation. This is likely influenced by the disciplinary orientation of participating scholars. Such discussions often seek to demonstrate or prove fairness as itself an algorithmic question, one rooted in questions of distribution. This is closer to ways of thinking about fairness within economics, but more distant from legal views or activist campaigns that align fairness more broadly with justice. In this talk, I will describe my own recent research to slightly expand the conversation outward beyond an inner circle of algorithmic fairness scholars, to include the experiences of people subject to algorithmic classification and decision-making. Using Twitter data, and specifically tweets about the “twitter algorithm,” I consider a wide range of concerns and desires Twitter users express. I find a concern with fairness (narrowly construed) is present, particularly in the ways users complain about the algorithms bias against particular groups. However, I find in collected tweet data another important category of concerns, reflecting a desire to enact more control over the algorithm. These tweets center consideration for human autonomy in the face of automation. In this talk, I will consider how questions of autonomy are implied in the framing of fairness, but also why they often end up being relegated to an ancillary concern. I will highlight some ways forward that respond to appeals for autonomy by those subject to algorithmic decision-making.
Human-Centered Data Science: Visual Interfaces for Making Sense of Data and Machine Learning
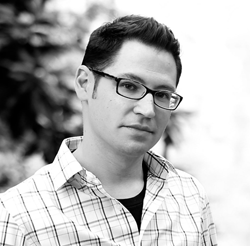
The ever growing volume of digital information has led to promises of a golden age for data analysis. While access to such data is paramount, access alone is ultimately insufficient without understanding the data’s patterns, identifying its outliers, and discovering its gaps. Data scientists often struggle to discover such insights in large and complex datasets without relying on automated machine learning approaches. However, purely automated techniques limit scientists’ abilities to explore data creatively or exploit their own domain expertise.
My research focuses on augmenting human intelligence by designing, building, deploying, and studying human-centered approaches to data science. I characterize my research as Human-Centered Data Science where I combine techniques from statistics, machine learning, and data visualization to empower data scientists throughout their analytical workflow. My talk will focus on three themes: designing tools for interactive and visual data science, providing data scientists with information and insights from interpretable machine learning and predictive modeling, and directly impacting a challenging and important domain with data-driven healthcare.