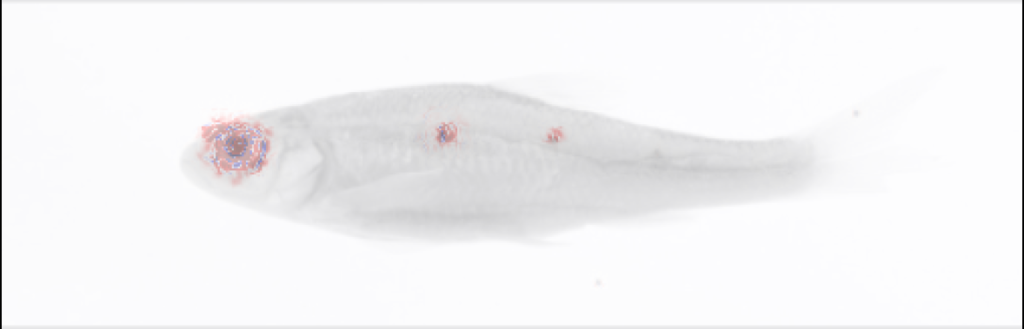
Taking butterflies and teleost fish as a case-study, this project tackles the problem of species modelling in the paucity of labelled training data.
As a solution, I propose taking taxonomic and phylogenetic information into account during model training. This modification improves the quality of learned features and their correlation to biological traits, leading to an improvement in the performance and robustness of downstream tasks, such as species classification.
Moreover, this project is concerned with obtaining interpretable models that can answer questions of interest to biologists, such as finding the phenotypical differences between a pair of species in a phylogeny tree or a co-mimicry relationship.