Summary:
Soylent, proposed by Michael S. Bernstein et al. , is a word processing interface that utilizes crowd contributions to aid complex writing tasks ranging from error prevention and paragraph shortening to automation of tasks like citation searches and tense changes. Soylent aids the writing process by combining Amazon’s Mechanical Turk workers and Microsoft Word. Soylent comprises of 3 components:
- Shortn
- Crowdproof
- The Human Macro
Shortn works on shorterning the request text down to 78%–90% of it’s original size. Crowdproof catches spelling and grammatical errors, with the accuracy of 67%. Human macro refers to custom requests made to the Mechanical Turkers. So far there has been 88% of intent success, which basically means 88% of the time the custom requests made to Turkers were understood correctly and was dealt with.
Soylent uses Find-Fix-Verify to make changes. They distribute the requests and first find the places that need attention. Then they apply Fix to those parts. Later they verified whether the fix applied was acceptable or not.
Reflection:
To me, the idea about crowd-sourcing one of the problems that still can’t be dealt with computers totally yet seems pretty novel. Though these days Google’s text prediction, spelling and grammatical error detection and reply to messages is pretty scary, but it’s still far from perfect. We still need to ask our co-workers, lab-mates, friends to assist us with proof-reading. So in that aspect, Soylent is an amazing idea.
While going through the paper, I was thinking that the whole idea is basically a bigger version of Wizard of Oz. My thought was pretty much verified with the line “Our work suggests that it may be possible to transition from an era where Wizard of Oz techniques were used only as prototyping tools to an era where a “Wizard of Turk” can be permanently wired into a system.” I really loved the idea of using crowd-sourcing to solve problem and later on use that to train Artificial intelligence, so that they can deal with similar problems in the future. I think the other technology that uses similar idea is Captcha.
The Lazy Turker and Eager Beaver problem seemed pretty interesting to me. We do seem to come across such problem in different settings. I really loved how they forced the lazy turkers to put more effort in their work. The usage of find-fix-verify definitely helped mitigating the problem of these cases.
One of the aspects I loved most about the paper was their conclusion. They actually showcased how Soylent works by shortening, proofreading their conclusion section using it. That’s pretty creative. I love it!
P.S: Through the paper this was going through my head and I HAD to add it here.
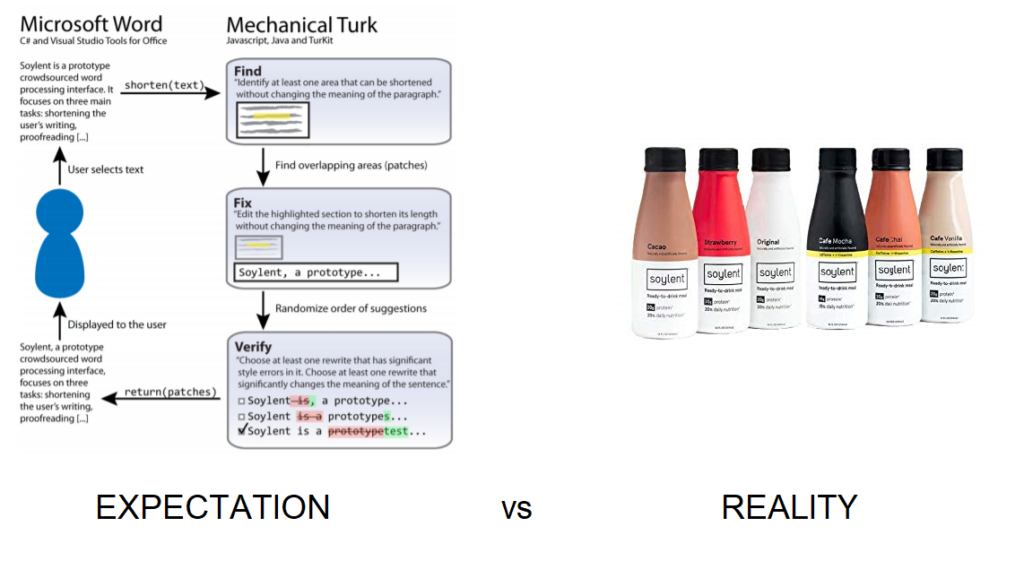