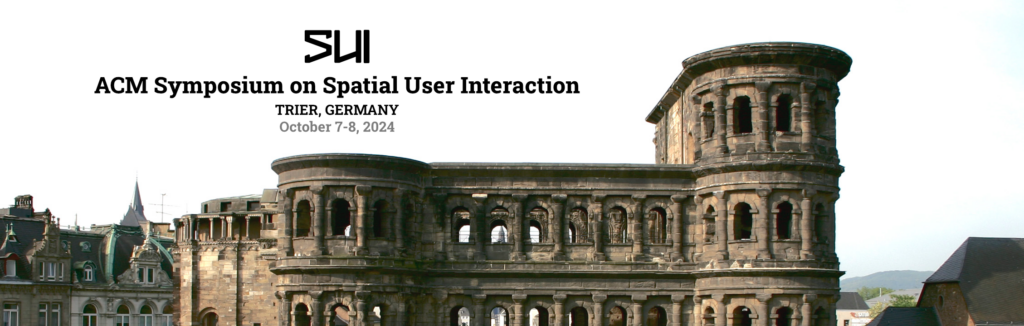
The 3DI group would like to take a moment to acknowledge the contributions of its members and alumni at SUI 2024.
Cory “Ike” Ilo presented his paper Goldilocks Zoning: Evaluating a Gaze-Aware Approach to Task-Agnostic VR Notification Placement. The abstract of the paper follows:
While virtual reality (VR) offers immersive experiences, users need to remain aware of notifications from outside VR. However, inserting notifications into a VR experience can result in distraction or breaks in presence, since existing notification systems in VR use static placement and lack situational awareness. We address this challenge by introducing a novel notification placement technique, Goldilocks Zoning (GZ), which leverages a 360-degree heatmap generated using gaze data to place notifications near salient areas of the environment without obstructing the primary task. To investigate the effectiveness of this technique, we conducted a dual-task experiment comparing GZ to common notification placement techniques. We found that GZ had similar performance to state-of-the-art techniques in a variety of primary task scenarios. Our study reveals that no single technique is universally optimal in dynamic settings, underscoring the potential for adaptive approaches to notification management. As a step in this direction, we explored the potential to use machine learning to predict the task based on the gaze heatmap.
Daniel Stover presented his paper TAGGAR: General-Purpose Task Guidance from Natural Language in Augmented Reality using Vision-Language Models. The abstract of the paper follows:
Augmented reality (AR) task guidance systems provide assistance for procedural tasks by rendering virtual guidance visuals within the real-world environment. Current AR task guidance systems are limited in that they require AR system experts to manually place visuals, require models of real-world objects, or only function for limited tasks or environments. We propose a general-purpose AR task guidance approach for tasks defined by natural language. Our approach allows an operator to take pictures of relevant objects and write task instructions for an end user, which are used by the system to determine where to place guidance visuals. Then, an end user can receive and follow guidance even if objects change locations or environments. Our approach utilizes current vision-language machine learning models for text and image semantic understanding and object localization. We built a proof-of-concept system called TAGGAR using our approach and tested its accuracy and usability in a user study. We found that all operators were able to generate clear guidance for tasks and end users were able to follow the guidance visuals to complete the expected action 85.7% of the time without any knowledge of the tasks.
Congratulations to both of the authors!