- Cristian Danescu-Niculescu et al. “A Computational approach to politeness with application to social factors”
The authors consider politeness in discussion and their impact on power dynamics. Using crowd-labelled data from StackOverflow and Wikipedia discussions, they are able to identify politeness markers and train a classifier with those markers towards automating the labeling process. They make the data (and the resulting tool) publicly available as a part of their contribution. The politeness tool, provides further insights about how the auto-labeling works, and how the use and placement of keyword affect the general tone of the sentence.
Interesting insights beyond what makes a post/question (im)polite, they are able to distinguish politeness by region (mid-westerners are more polite), by programming language (Python programmers are the most impolite, Ruby the most polite) and gender (women are unsurprisingly more polite) — these findings serve to ground their research on a platform-independent way.
Balanced vs Power Dynamics
The major consideration of this work was in probing power dynamics, by looking at the imbalance between administrators and normal users. It would be very interesting to extend this to note the general tone of users in a balanced discourse: If there are no explicit rules in a forum on how to conduct a discussion, is there an imbalance on conversation? Does it impact the willingness of users replying if the original question is impolite? Using the same classifier to categorize these new discussions would be a straightforward step.
Bless your heart: Sarcasm and other language features
The figure below showcases aspects that are not considered by the classifier: namely sarcasm and colloquial terms. This is expected, as no classifier is perfect except with constant learning. The politeness tool also provides a means for the user to relabel the sentences according to whether they agreed on the label (and confidence) or not. Presumably there is a mechanism to improve the classifier’s prediction accuracy by having this human-in-the-loop provision. It does not impact the paper’s contribution and impact, but it continues to raise the general question on the efficacy of machine language in understanding human language.
Beyond Wikipedia and Stack Overflow
Is bot-speak polite? And are there languages markers that distinguish them from a typical user? The use of such markers would serve as a useful augment to current means of identifying bots that rely on profile features and swarm behavior. This knowledge can further be used in strengthening spam filters on places such as discussion under articles against posts with ‘bot-like’ languages.
Do users care whether their language is considered impolite? Beyond a would-be Wikipedia editor using the knowledge gained about the impact of politeness on their chances of being granted the post, does a questioner on StackOverflow or any other platform with a clear power imbalance care that their tone is impolite? Does the respondent (or the original questioner)? Or do they only care that the answer has been received? Further behaviors can then be discussed about whether the change of behavior is successful towards getting the user their desired goals, or whether the gains do not depend on the user. In which case factors such as personality — the fact that successful would-be Wikipedia administrators for example had a predilection towards the leadership position that cannot be explained by language alone (or lend them towards utilizing language skills towards getting the rewards).
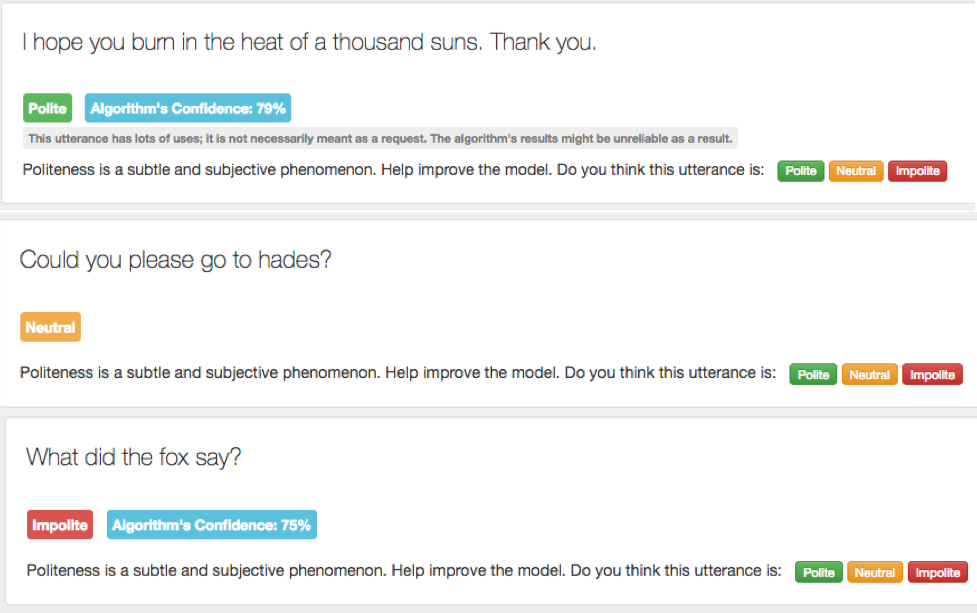
One thought on “Reflection 11 – [10/11] – [Lindah Kotut]”